主講人:李奕霈教授 (國立臺灣大學化學工程系)
題目:The Good and the Bad of Deep Learning for Molecular Property Predictions
時間:111年10月27日(四) 18:00
報名網址: https://forms.gle/PyynQugak4dqb5N38
報名截止時間 :111年10月27日中午12點止
報告語言: 中文
大綱:
Recent advances in deep neural networks have led to the development of models with remarkable accuracy for molecular property predictions and have successfully facilitated the development process in various fields, including retrosynthesis, drug design, and reaction engineering. Although deep learning has been shown to perform well in many applications, its accuracy and applicability strongly depend on the quality and quantity of available data. Since most of the research is carried out with a clear goal, data reported in the literature usually focus on certain regions of the chemical space. Therefore, in the field of chemistry, the accuracy of data-driven models is not always satisfactory, especially in new research areas where data are scarce. For this reason, assessing when and to what extent a prediction can be considered reliable is an important task for applying deep learning to the prediction of molecular properties. In this presentation, we will take the prediction of molecular thermochemistry as an example to discuss how one can deliberately design the architecture of deep neural networks to improve model generalizability and leverage active and transfer learning strategies to reduce the amount of data required. The prospect of combining deep learning models with scalable uncertainty quantification methods to derive explainable and calibrated uncertainty for molecular property predictions will also be discussed.
References
- Li, Y.-P.; Han, K.; Grambow, C. A.; Green, W. H. Self-Evolving Machine: A Continuously Improving Model for Molecular Thermochemistry. J. Phys. Chem. A 2019, 123 (10), 2142–
2152.
https://doi.org/10.1021/acs.jpca.8b10789.
- Scalia, G.; Grambow, C. A.; Pernici, B.; Li, Y.-P.; Green, W. H. Evaluating Scalable Uncertainty Estimation Methods for Deep Learning-Based Molecular Property Prediction. J. Chem. Inf. Model. 2020, 60 (6), 2697–2717. https://doi.org/10.1021/acs.jcim.9b0097.
- Chen, L.-Y.; Hsu, T.-W.; Hsiung, T.-C.; Li, Y.-P. Deep Learning-Based Increment Theory for Formation Enthalpy Predictions. 2022. https://doi.org/10.26434/chemrxiv-2022-lc84d.
- Yang, C.-I.; Li, Y.-P. Explainable Uncertainty Quantifications for Deep Learning-Based Molecular Property Prediction. 2022. https://doi.org/10.26434/chemrxiv-2022-qt49t.
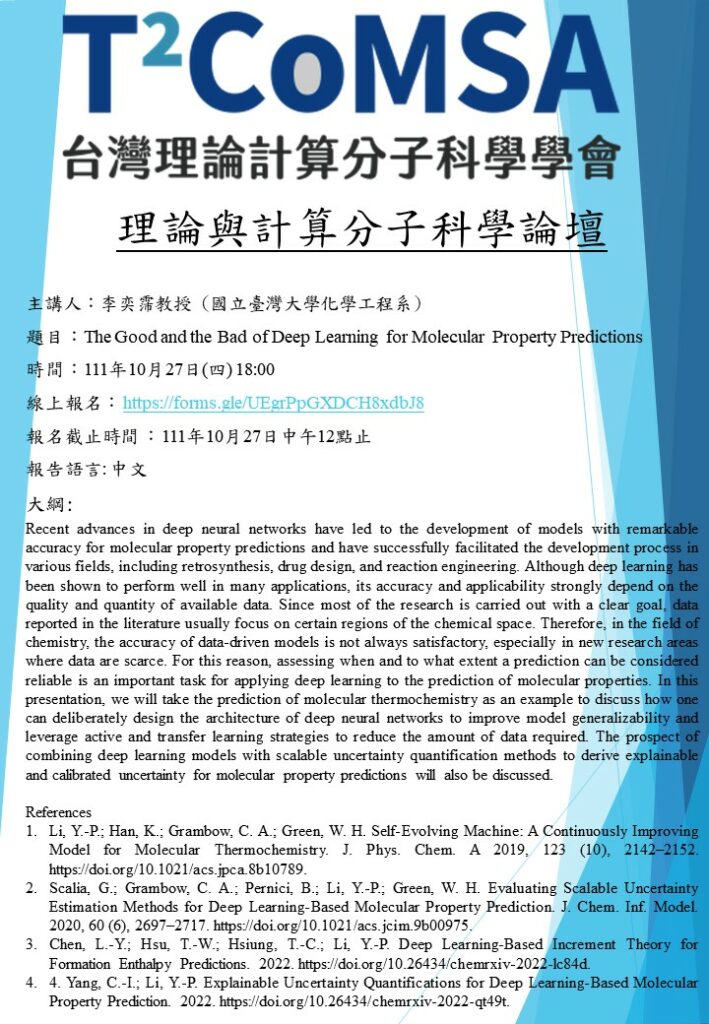